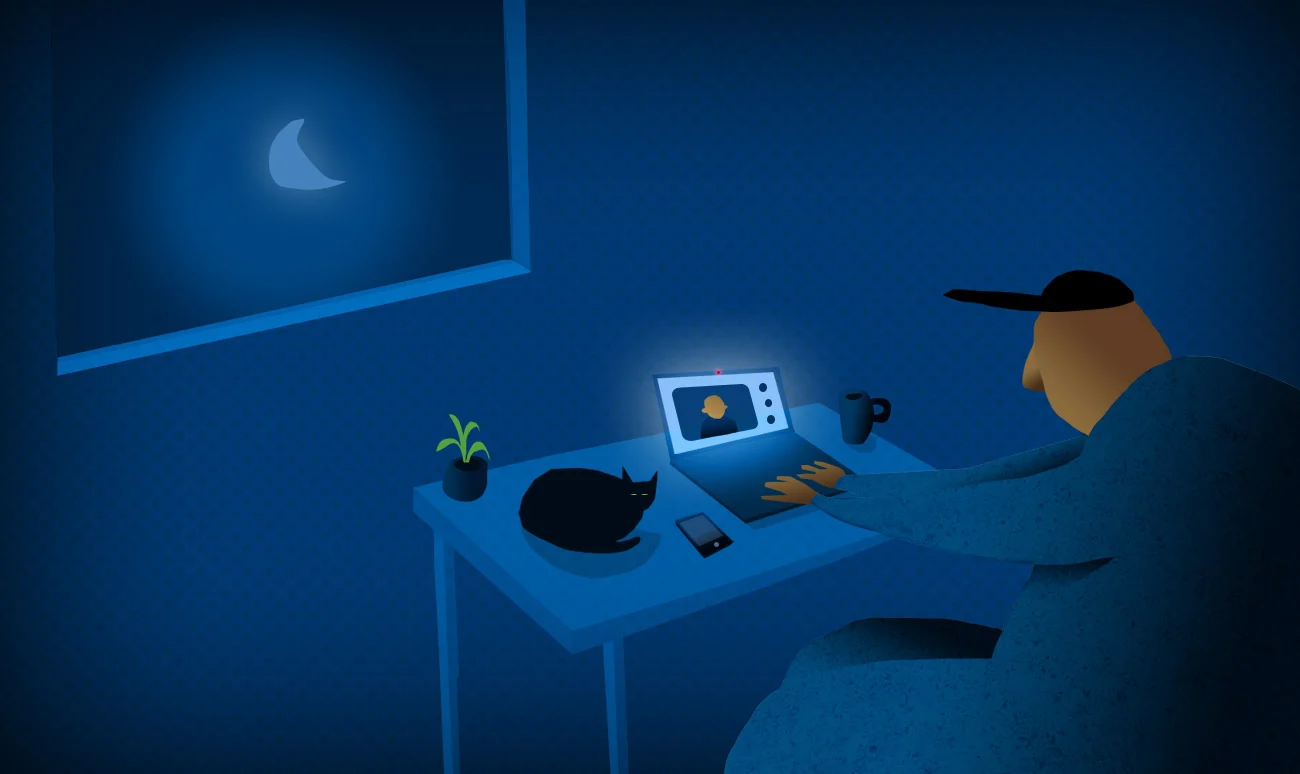
Illustration by Benjamin Westerfjell
When you think about online exams, what are the benefits that first come to mind?
It’s likely that the ability to hold exams remotely is one of them. After all, with widespread college closures and a growing student appetite for flexible learning, online exams increasingly feature in higher education assessment strategies.
But, while the ability to offer remote exams is certainly important, it’s not the only benefit of online exams. In fact, some Inspera customers choose to hold online exams on site but still see a number of advantages in comparison to traditional assessment methods. Let’s have a look at a few of the benefits of online exams beyond flexibility.
Alignment Between Teaching and Assessment Tools
Many institutions around the world use digital tools in the classroom. Whether sharing resources through an online library, collecting coursework submissions via a virtual learning environment(VLE)/learning management system or setting practical tasks in specialist software applications, technology is the norm for students today.
It makes sense, then, that students are assessed in the same way that they learn. After all, students don’t often handwrite their work during the rest of the year. Asking them to do so during a summative exam may add additional pressure to an already stressful time.
Reinforcement of Digital Skills
Similarly, the tools of many modern trades are now digital. An increasing number of career paths have sprung entirely from the digital revolution including software development, digital marketing and data science to name a few. Yet even careers as old as time, from teaching to farming, require digital skills.
Using a digital assessment platform allows students to reinforce these skills in a number of different ways. The first is that it inherently requires basic digital skills, such as word processing and information retrieval, which are essential for life post education. Exam authors can go one step further and incorporate industry-specific tools or resources into an exam or assessment so that learners can showcase their skills in real-world scenarios.
Efficient Examination Processes
One of the key online exam benefits that users may notice is an increase in efficiency. If you currently use paper exams, you’ll know only too well the time-consuming process of booking exam venues, organizing printing and transporting papers. And that’s before even considering authoring the exam, preparing candidate lists, arranging exam proctoring, grading submissions and sharing feedback.
Although digitalizing your assessments won’t make all of these elements go away, it can significantly reduce the logistical tasks associated with delivering paper exams. Because everything takes place on-screen, time is saved transporting exams to venues and then re-distributing to graders, for example. Plus, a single digital assessment platform provides an easy way for planners, authors and graders to collaborate without needing to use multiple documents. The University of Gothenburg, who have been working with Inspera since 2015, have saved 4-6 hours on the administration process of each exam in some cases.
Greater Transparency
Another key benefit of online assessment is the amount of transparency it affords both university staff and students. When everything is in one place, it’s easier to get a clear overview of each part of the process. During the exam, proctors have an overview of how each student is progressing and can see how long it has taken to complete the test. Afterwards, administrators can see how many exams have been marked, allowing them to follow up with markers if necessary. Perhaps best of all, staff can completely forget the worry of lost exam papers. Plus, with all examination data stored in a single system, dealing with student appeals is much quicker and more efficient.
Reduced Environmental Impact
An Australian university previously held 360,000 exams every year which used 8.5 million sheets of paper, equivalent to 1,000 trees (source). This is before taking into account the transportation required to get exam scripts to venues and back. When exams take place digitally, it’s possible to significantly reduce this impact. According to Donald Lancaster, Senior Lecturer at The University of Bath, 2 hours of laptop usage would only be about 0.00007-0.00020 kg CO2 in comparison (source).
If online exams are here to stay, institutions can expect to see a variety of benefits for students, staff and the planet. If you’d like to hear more about the long-term advantages of online exams, tune in to episode 2 of The Digital Assessment Podcast with Suzanna Doran and Ishan Kolhatkar. You can find all of our new episodes here as well as on Spotify, Apple Podcasts and Google Podcasts.
Frequently Asked Questions About the Benefits of Online Exams
Do Students Perform Better in Online Exams?
Academic integrity is often cited as a reason for the reluctance to introduce or continue with online exams on a more permanent basis. While institutions may be wary of online exams providing easier access to student plagiarism or cheating, this does not appear to have resulted in unnatural spikes in student performance. Online exams can be applied in a variety of different ways to maintain both academic integrity and a consistent way of measuring student performance.
Mario Micheli, a senior lecturer in Applied Mathematics and Statistics at John Hopkins University carried out online exams post-Covid without smarter proctoring. But he changed his usual form of testing from closed-book to open-book assessment. He commented that the change in assessment had not affected grade distribution across the course:
“It seemed to work quite well because it was timed — the students were so focused for such a short period of time that it would have been a waste of time to talk to others,” he said (source).
There has also been pre-pandemic research on whether students test better on paper or computer, such as that carried out by the Fundamentals of Engineering for Honors (FEH) program at The Ohio State University (2014). Their study found no significant differences between test scores conducted on computer vs paper for first-semester undergraduates on the FEH program.
Similarly, a study carried out by Karay, Schauber, Stosch & Schüttpelz-Brauns (2015) on German students in their ninth and tenth semester of medicine (comparable to the fourth-year of North American medical school) found no main differences in test scores between matched pairs completing computer exams and paper exams. However, they did note that students managed to complete the computer exam in significantly less time compared to the paper exam. This they attributed to less processing time needed for computer exams compared to paper exams.